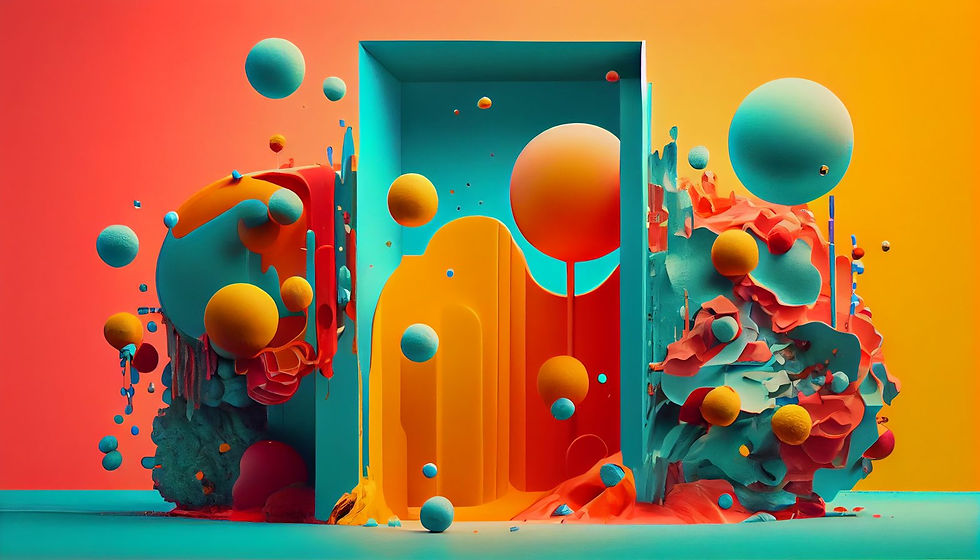
Chelonia is currently engaging in the scouting of experts and stakeholders eager to contribute to new initiatives and collaborative projects with our pharma partners.
In line with this effort, today we delve into the topic of generative AI. This innovative field leverages advanced algorithms to create content and solve problems autonomously, representing a cutting-edge frontier in technology development. As Chelonisa explores potential collaborations, the focus on generative AI highlights the company's commitment to embracing emerging technologies that drive progress and innovation.
Generative AI is catalyzing a transformation in the pharmaceutical industry, bringing about substantial efficiencies and innovations across the drug development lifecycle. This technology is estimated to create $60 billion to $110 billion in annual economic value by enhancing various aspects of the sector, from research and discovery to commercialization.
Generative AI can significantly speed up the process of identifying viable drug candidates. By integrating deep learning models like AlphaFold2 and ESMFold, AI can predict the structures of almost all known proteins, offering new insights into their functions and related diseases. This capability allows for quicker identification of targets for new drugs, potentially reducing the timeline for drug discovery.
AI-driven tools streamline the clinical trial process, making it more efficient and effective. They can predict patient responses to treatments, thereby optimizing trial design and patient selection. This not only improves the quality of clinical trials but also reduces their duration and cost. For instance, AI can analyze vast amounts of real-world data to identify potential trial participants who are more likely to respond positively to a treatment, thus increasing the success rates of clinical trials.
In the operational realm, generative AI introduces automation in areas such as document processing, regulatory submissions, and compliance checks. These applications reduce manual workloads, cut down processing times, and enhance accuracy. For example, AI can automatically generate first drafts of clinical-study reports and regulatory documents, which are then refined by human experts.
AI also transforms how pharmaceutical products are marketed and sold. It enables personalized marketing by analyzing patient data to create targeted marketing strategies and materials. Moreover, AI tools can help sales teams by providing them with detailed customer insights and optimized engagement strategies.
Despite these benefits, implementing generative AI in the pharmaceutical industry comes with its challenges. These include ensuring data privacy, managing integration with existing systems, and addressing regulatory concerns. Companies must also focus on scaling these solutions while maintaining accuracy and reliability.
The adoption of generative AI in the pharmaceutical industry represents a significant shift towards more data-driven, efficient, and personalized medicine. However, success requires careful consideration of technological, regulatory, and ethical standards to fully realize the potential of AI in improving patient outcomes and operational efficiencies.
Generative AI in Drug Repurposing: An Accessible Explanation
Drug repurposing involves finding new uses for existing drugs, offering a cost-effective and time-efficient alternative to developing new medications from scratch. This approach is particularly valuable for diseases like Alzheimer's, where new effective treatments are urgently needed. Generative AI (GAI), such as OpenAI's ChatGPT, uses large amounts of data to generate new content and ideas. In the context of drug repurposing, GAI can analyze extensive scientific literature to identify potential new uses for existing drugs.
How Does GAI Work in Drug Repurposing?
Data Input: ChatGPT, for instance, has been trained on a vast array of scientific papers, medical research, and other relevant data.
Query Processing: Researchers can ask GAI tools specific questions like "What existing drugs could potentially be repurposed for treating Alzheimer’s disease?"
Generating Hypotheses: The AI analyzes its training data to generate a list of drugs that might be effective against Alzheimer’s, based on their mechanisms of action, effects observed in related conditions, or evidence of efficacy in unexpected contexts.
Researchers use GAI to sift through data and suggest drugs that might reduce Alzheimer's risk or symptoms. They test these suggestions using real-world data (like patient records from Vanderbilt University Medical Center) to see if people taking these drugs for other reasons have a lower incidence of Alzheimer's.
Benefits of Using GAI ?
Speed: GAI can process thousands of documents in minutes, much faster than humans.
Cost-Effective: It reduces the need for initial expensive experimental testing by narrowing down the list of potential drugs.
Up-to-Date: AI tools continuously learn from new data, keeping their suggestions relevant.
Real-World Impact ?
In a hypothetical study, drugs like metformin and losartan were suggested by GAI and then found to potentially lower Alzheimer's risk in older adults through analysis of clinical data.
Generative AI has the potential to revolutionize drug repurposing by quickly identifying promising candidates from existing drugs.